Home Blog Criteria for Evaluating Procedure Analytics in Supply Chain
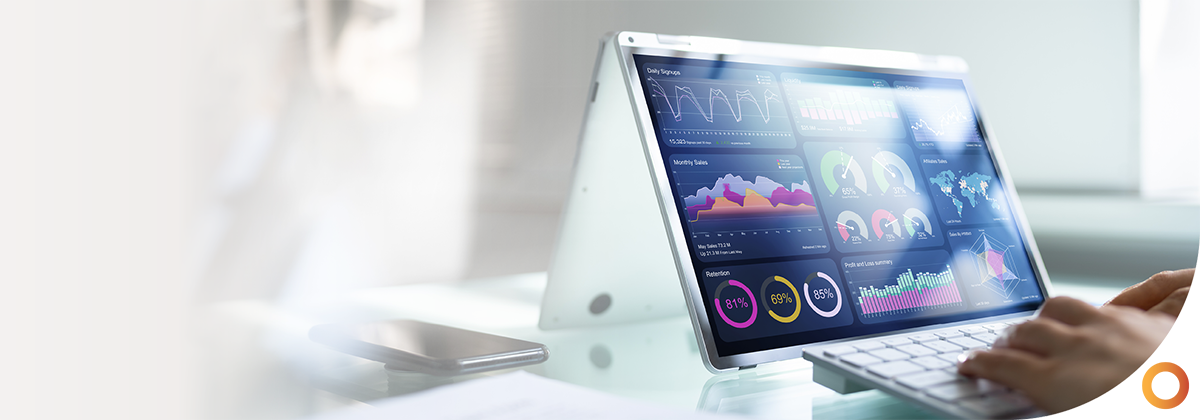
Criteria for Evaluating Procedure Analytics in Supply Chain
Procedure analytics programs are used by health systems to help support a broader clinical integration initiative between the supply chain team and clinicians to drive value to patients in terms of quality care and outcomes. To create a successful collaboration and for the supply chain team to have solid conversations with clinicians, the health system needs reliable information around clinical products and constructs to fully comprehend the quality impact. Clinicians have high expectations for these teams to have a thorough understanding of how clinical products and components come together into the construct in any given case, and how it impacts the value equation and outcomes.
Through procedure analytics, health systems aspire to move beyond measuring practice patterns for savings and standardization opportunities. Instead, encouraging broader practice adoption across all clinicians, and be able to circulate accurate and relevant data on an ongoing basis to realize opportunities for utilization and operational gains. Furthermore, health systems want to introduce advancing technology into the patient value equation. As such, when clinicians are introducing advancing technology that has had a positive impact on a patient’s value equation, health systems should then share this information in order to gain trust and buy-in. Currently, health systems may be on any side of the continuum – either working on operational impact and price savings, focused on standardization opportunities, or in a more mature stage of focusing on utilization changes and adoption of advancing technology.
Why do providers struggle with procedure and healthcare analytics solutions?
Before discussing decision criteria for vetting procedure analytics solutions, it is important to recognize why providers struggle with successful implementations. When teams initially invest in procedure analytics solutions, the data is often presented through appealing visuals and in a way that supply chain and clinical teams understand it as part of the organizational vision. However, once these teams begin to implement the solution, it often breaks.
For example, teams are realizing that the data is not classified in a way that is usable, which presents a significant data problem as this requires a significant amount of manual work for the solutions partner to organize data into meaningful insights. When these partners quote the health system, this quote only encompasses the software, when in reality, the software does not solve the clinical data problem. This poses a frustrating issue as the health system originally accounts for a software spend and expects to transition through the maturity curve and implement procedure analytics in their health system, but does not account for the spend in analyst resources that are required to fix these clinical product data problems. This issue is a huge opportunity cost as it either requires someone on the team to be pulled from other important projects to manually resolve these data issues, or health systems need to spend significant unbudgeted investments to solve the problem with external analyst or consultant resources. For this reason, it is exceedingly difficult for health systems to reach a future state with procedure analytics as it becomes cost prohibitive in terms of both time and money. Ultimately, due to this high cost and effort, health systems must abandon utilization tracking in major clinical categories and continue down the path of only focusing on large savings projects every 18-24 months, when the patient value equation has been so vastly impacted that it can no longer be ignored.
How can health systems better vet a procedure analytics solution?
Health systems spend a significant amount of money trying to solve clinical product data accuracy challenges. Many GPOs and software vendors tend to distract with the promise of fancy visuals and clinical “experts” rather than directly solving the clinical product data accuracy with powerful classification and automation. As these data problems are such a costly problem, consider the following criteria:
- What classification system is being used to drive this information? Ask for detailed breakdowns on how multidimensional the classification is. Hint: if it is using UNSPSC, this is not multidimensional and therefore cannot handle the specificity needed for analyzing clinical parts and products. For example, if you need to run a category-specific, construct-specific, or implant-specific report to support a savings initiative and clinical presentation, there will be irrelevant parts showing up that should never be there. Your team needs a multidimensional classification system that can differentiate clinical products – i.e., coated vs. uncoated or primary vs. revision. If your team does not clarify which classification system is being used, there is a risk of spending money on a procedure analytics technology that produces data that clinicians do not trust.
- Is there an ability to break the data out into different parts? It is essential for your team to be able to see what data is being grouped together. For example, is there a way to split orthopedic trauma data from CMF? Can you isolate data when the parts are not classified correctly? What are the high-level data match rates? Implant data should be matched at a rate of 95% or higher through automation without manual intervention. As part of these evaluations of procedure analytics, there needs to be a data analyst on the team that is willing to audit and evaluate the quality of the underlying data being used to support the visuals.
- When evaluating procedure analytics, how much budget do I need to allocate on an annual basis for data analyst support? Look at the turnaround times on data as this tells you how much manual work is being put in to solve for these challenges. As such, it is important to know what percent of time and data was driven by artificial intelligence and machine learning compared to the percentage that was driven manually. In order to vet the power of automation (which is where providers see efficiency gains that make it fiscally possible to maintain consistent reporting and monitoring), the health system should ask for a quick turn of a test data set which should be delivered in a matter of hours or days, not weeks. Additionally, the amount of time and spend needed for an analyst to correct clinical product data accuracy challenges should be realized prior to vendor selection instead of a year down the road to ensure it is part of the budget discussion with the technology vendor. That helps to confirm if it is fiscally reasonable to consistently produce these reports.
- Does the procedure analytics solutions automatically break down constructs at a component level stock-keeping unit (SKU), and compare benchmarks based on an exact match of those catalog numbers?
These solutions should also be able to differentiate construct and component utilization by a physician, procedure type, and vendor, as well as be able to rebuild this level of reporting on a consistent basis. Regardless of whether or not the health system uses a constructed strategy, component strategy, or uses a matrix approach, they need to be informed of benchmark savings opportunities at both the construct and component level.
Clinical product data analysis questions?
Getting to the core of the problem and getting good data to earn trust among clinicians is key when vetting procedure analytics solutions for your health system to implement. Choose a partner and procedure analytics solution that can help your health system grow and achieve its broader goals of clinical integration and patient value. Do you have questions on your organization’s data analysis procedure? Put Curvo’s data to the test. Contact our team to schedule an instant match on an orthopedic vendor file, and schedule a demo of Curvo for free.