Home Blog Data Management Glossary for the Healthcare Supply Chain
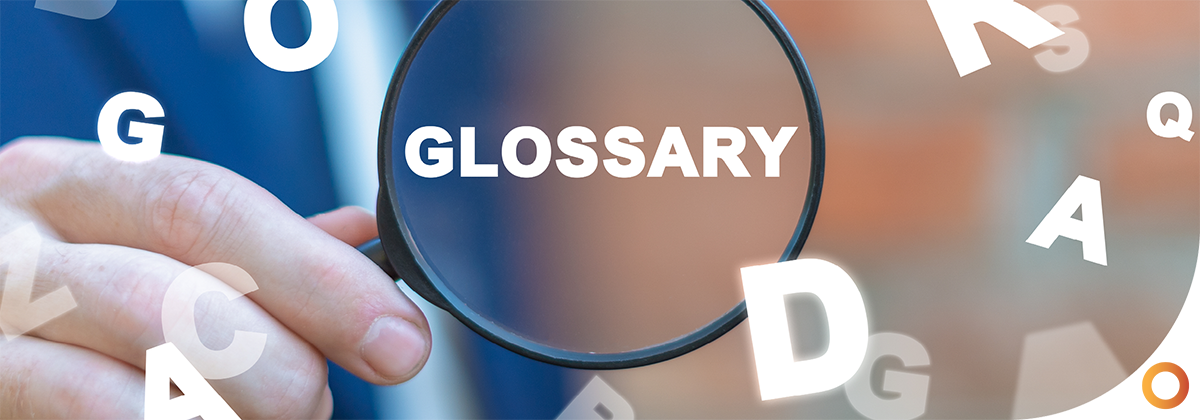
Data Management Glossary for the Healthcare Supply Chain
Data Enrichment & Benchmarking Supply Chain
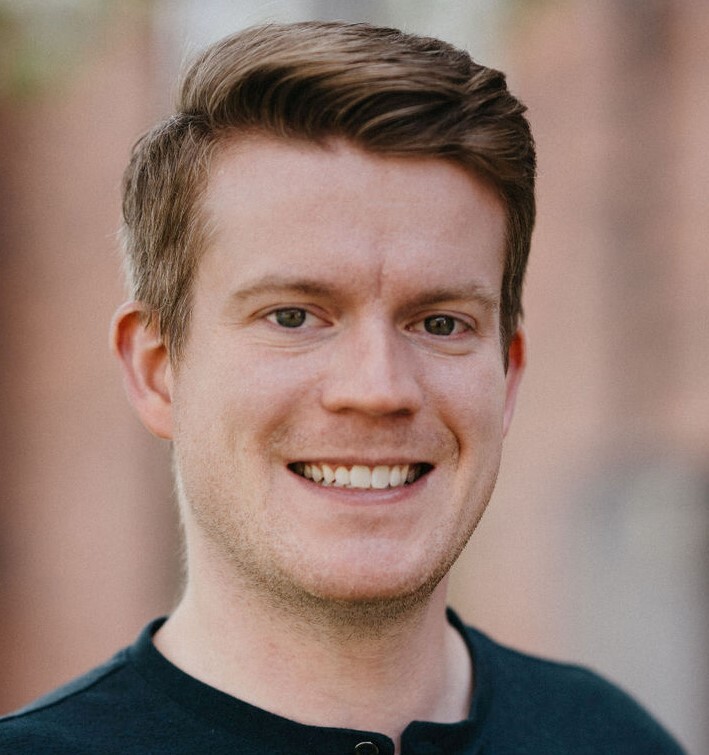
Greg Prince
Editor’s Note: Curvo Director of Data Enrichment, Greg Prince, contributed to the data glossary. A Vanderbilt graduate (BA and MBA), Greg has a deep background in healthcare supply chain as a consultant and in leadership across multiple service lines. Today he heads up Curvo Data Operations, providing data analytics and management for dozens of health systems. His team is responsible for acquisition and throughput of data and corresponding normalization and enrichments that help hospitals optimize spend.
It’s easy to get confused by all the similar terms used in the realm of data management, and no wonder. Even the 60+ data terms and definitions we’ve collected here isn’t a complete list of what the healthcare supply chain might encounter. The world of medical product data changes constantly and takes work to keep up with normalizations, enrichments, terminology and more.
We understand the importance of data and want to help educate others who need to know about this for data-driven sourcing. There’s a lot to know about data management, and these terms and definitions might help when you want to learn more about key aspects of your data practices.
60+ Data Management Terms for Supply Chain Reference
If you’re transforming into a more data-driven supply chain, you need to be fluent in the language. Here are data-related terms for the data-driven healthcare supply chain.
- Data Analytics - Data analytics in the healthcare supply chain involves the systematic examination of data to extract valuable insights, optimize inventory management, enhance distribution efficiency, and improve decision-making processes to ensure the timely and cost-effective delivery of medical supplies and equipment to healthcare facilities.
- Data Appending - Data appending refers to the process of enhancing and updating existing datasets with additional information, such as clinical details, product specifications, or supplier information, to improve the accuracy and completeness of existing supply chain records.
- Data Architecture - Outlines the structure for flow of information within an organization, encompassing both physical and logical data assets. It establishes control mechanisms and aims to translate business requirements into data and system specifications, while overseeing the management and movement of data throughout the enterprise.
- Data Augmentation - Method employed to artificially expand the volume of training data derived from existing datasets, without the necessity of gathering fresh data. Data augmentation refers to enhancing or expanding existing data sets by techniques such as image manipulation, text generation, or synthetic data creation, particularly in the context of the healthcare supply chain, to improve the quality and quantity of data for analysis, forecasting, and decision-making.
- Data Availability - Data availability in the healthcare supply chain refers to the continuous and reliable access to essential data and information necessary for efficient management of medical inventory, ensuring that healthcare facilities have the right supplies when needed to provide patient care.
- Data Bottleneck - A step in the data pipeline that slows down or restricts throughput of data to end users. A data bottleneck in the healthcare supply chain refers to a situation where information flow is constrained or delayed, impeding efficient management and distribution of medical supplies, resulting in potential disruptions and inefficiencies.
- Data Catalog - A data catalog serves as a comprehensive inventory of an organization's data assets, utilizing metadata to expedite discovery, access, and assessment of the most suitable data for analytical or business purposes.
- Data Classification - Data classification in the healthcare supply chain involves categorizing and labeling data to prioritize its handling, ensuring sensitive patient and supply chain information is appropriately protected and managed based on its sensitivity and regulatory requirements. Data classification using medical device data is the process of categorizing and labeling data to identify its sensitivity, ensuring proper handling, access controls, and compliance with regulatory requirements for the secure and efficient management of medical device-related information.
- Data Cleansing - Process of preparing data for analysis by rectifying or eliminating erroneous, corrupted, improperly structured, duplicated, irrelevant, or incomplete data within a dataset. Data cleansing in clinical spend management analysis refers to the process of identifying and rectifying errors, inconsistencies, and inaccuracies in financial and procurement data. The goal is to ensure the integrity and reliability of cost analysis and supply chain optimization.
- Data Curation - Includes activities like data integration, selection, classification, transformation, validation, and preservation. Data curation in the context of medical device data in the healthcare supply chain involves the systematic organization, validation, and maintenance of relevant information. Hospitals can maintain the accuracy and accessibility of critical data for supply chain spend management, contract compliance and care quality.
- Data Democratization - Data is made accessible to the average user without barriers or gatekeepers impeding access. In the healthcare supply chain, this refers to the process of making critical data and information accessible and understandable to a broader range of stakeholders, including clinicians. Data democratization promotes informed decision-making and collaboration with supply chain, clinicians, and administration, ultimately improving its data efficiency and effectiveness.
- Data Efficiency - Data efficiency in the healthcare supply chain refers to finding optimal use of data and information systems to streamline processes, reduce waste, and improve overall effectiveness of acquiring and managing clinical devices. It accounts for throughput of data from Point A to Point B, while identifying and addressing both data bottlenecks and data silos.
- Data Engineering - Data engineering in the context of clinical spend management analysis and the healthcare supply chain relates to collecting, transforming, and organizing data from various sources to support efficient cost analysis, pricing optimization, and spend management. Focuses on identification of sources, collection, curation, and storage.
- Data Enrichment - Process of enhancing, supplementing, refining, and improving collected data through incorporation of pertinent third-party data. Data enrichment teams add new and supplemental information as well as verify against third-party sources. They improve existing information by adding missing or incomplete data. In the context of clinical spend analysis, it refers to augmenting existing data with relevant information to gain insights into cost management and contract optimization.
- Data Exchange - Conversion of data from one file or database format into a format suitable for the target schema. In healthcare supply chain, this refers to seamless transfer and sharing of information like inventory levels, orders, and product tracking among stakeholders, including clinical leaders and physicians, suppliers, supply chain, and healthcare leaders to optimize clinical contracts.
- Data Export - Involves retrieving and transferring data with intent to process it in some way. Data export in the healthcare supply chain involves transferring relevant information or records, such as purchase orders or usage trends from one system or organization to another, often to support efficient sourcing workflow and spend management.
- Data Extensibility - Ability to expand and enhance an application with data from external sources, such as other applications, databases, and unique datasets. In the context of medical device data, it refers to the ability to easily augment, customize, or add new fields and attributes to an existing dataset. This allows integration and adaptation of diverse sources to meet specific supply chain needs and standards.
- Data Extraction - Data extraction is the process of accumulating or retrieving data from various sources for subsequent processing, storage, or analysis elsewhere. Data extraction in the context of using clinical product data in the healthcare supply chain refers to the process of retrieving relevant information from various sources, such as electronic health records or databases, to enable efficient inventory management, procurement, and distribution of medical supplies and products.
- Data Governance - Data governance in the healthcare supply chain involves establishing policies and procedures to ensure the accuracy, consistency and security of data related to healthcare expenses and supply chain operations, facilitating decision making, and usage compliance. Encompasses the framework of individuals, procedures and technologies required to manage, organize, and safeguard a hospital's clinical data assets.
- Data Health - Pertains to the condition of a company's data and its ability to support business objectives. Data health in the context of using clinical product data in the healthcare supply chain refers to the accuracy, consistency, and reliability of the information, ensuring that it is up-to-date and error-free to support effective decision-making and supply chain operations.
- Data Hygiene - Entails the continuous procedures employed to ensure that data is clean and ready for use. Data hygiene in the context of the healthcare supply chain refers to the process of maintaining and ensuring the accuracy, quality, and consistency of data used for managing and optimizing the supply of healthcare-related products and services.
- Data Import - Denotes the process of transferring data from external sources to another application or database. Data import in the healthcare supply chain refers to the process of transferring and integrating external data, such as inventory levels, demand forecasts, or order information, into the system for analysis and decision-making to optimize the distribution of medical supplies.
- Data Ingestion - Involves movement of data from multiple sources into a centralized database, typically a data warehouse to facilitate access and analysis in real-time or batch processing. In the healthcare supply chain, this refers to collecting and importing relevant data from various sources, such as suppliers and hospital facilities. This becomes a centralized system for efficient analysis and informed decisions.
- Data Integration - Consolidation of data from diverse sources to achieve unified, coherent views. In the healthcare supply chain, refers to combining and harmonizing data from various sources within healthcare system and third parties to ensure seamless, efficient management of clinical supplies spend.
- Data Integrity - Refers to overall accuracy, consistency, reliability of data throughout its lifecycle. In the healthcare supply chain, it refers to accuracy, consistency, and reliability of clinical product information, ensuring that data remains complete and unaltered throughout its lifecycle to support efficient and safe supply chain operations.
- Data Intelligence - Analyzing different data forms to enhance hospital services or purchase. In healthcare supply chains, data intelligence refers to systematic gathering, analysis, and application of data to optimize efficiency, transparency, and decision-making for medical device contracts.
- Data Interoperability - Ability of distinct IT systems and applications to create, exchange and consume data to facilitate use of exchanged information. Data interoperability in supply chain relates to seamless exchange and integration of data between different systems and stakeholders for efficient, accurate information sharing in support of the flow of medical products and services.
- Data Lake - Centralized storage repository that retains extensive data in its natural, unaltered format. A data lake in the healthcare supply chain context is a centralized repository that stores vast amounts of raw and unstructured data from various sources, enabling efficient data storage, management, and analysis for improving supply chain operations and decision-making.
- Data Management / Master Data Management - Data management in the healthcare supply chain involves the organized collection, storage, analysis and utilization of data. Good practices ensure efficient, accurate tracking of clinical product pricing, usage, and compliance. Solid device management ultimately improves overall supply chain effectiveness and reliability.
- Data Manipulation - Denotes process of structuring data to enhance readability or organization. Data manipulation in the clinical products supply chain refers to organizing, transforming, and processing data to optimize efficiency of managing the flow of medical device information.
- Data Mapping - The procedure of aligning data fields from one or more source files with data fields in another source. Data mapping in the context of spend management refers to systematically correlating and connecting various data sources and formats to facilitate efficient tracking and analysis of usage, contracts, bids and more throughout the clinical supply chain.
- Data Migration - Process of relocating internal data between file formats, databases, or storage systems. In clinical sourcing, data migration involves transferring, transforming, and updating data from one system or location to another. The goal is seamless exchange of information for efficient functioning within the clinical supply chain.
- Data Mining - Data mining in the context of clinical spend management refers to the process of analyzing large datasets to uncover valuable insights and patterns. These can be used to optimize cost-efficiency, resource allocation and sourcing strategies in the healthcare supply chain. The process uncovers anomalies and correlations within large data volumes to answer questions through analysis.
- Data Modeling - Process of visually representing data elements and their interrelationships. Healthcare supply chains use data modeling to create structured representations of data that helps them understand, organize, and optimize the flow of high-ticket medical devices. PPI contracts benefit from informed decision-making and efficient resource management.
- Data Normalization - Takes data standardization one step further by making data less vague and more usable across business systems. Healthcare supply chains use the practice to standardize and structure financial and product data needed for clinical spend management. Supply chains can provide accurate analysis and comparison of medical products, helping hospitals make informed contract decisions, control variations, and reduce costs.
- Data Onboarding - Process of incorporating clean, external data into sourcing applications and operational systems. In clinical spend management, it refers to importing and integrating financial and procurement data from various sources, such as invoices and purchase orders. This goes into a centralized system for analysis and optimization of implant-related expenditures.
- Data Orchestration - Aggregation, combination, and organization of data to render it accessible to data analysis tools. Data orchestration in clinical spend management refers to the process of efficiently collecting, aggregating, and harmonizing medical device data from various sources. Contributes to better decision making and cost optimization for medical device spend control.
- Data Pipeline - The series of steps necessary to transfer data from one system (source) to another (destination). A data pipeline in the healthcare supply chain is a structured, automated process for collecting, processing, and disseminating data from various sources. Astable pipeline allows efficient clinical spend management.
- Data Portability - Signifies ease of transfer and use of data among different applications, programs, computing environments, and cloud services. Data portability in the healthcare supply chain refers to the seamless transfer and accessibility of financial and procurement data across hospital organizations, suppliers, and systems. Portability supports efficient cost analysis and contract decisioning.
- Data Privacy - As a component of data security, privacy concerns proper management of data in accord with consent, notice and regulatory obligations, Privacy practices emphasize compliance with data protection regulations.
- Data Quality - Denotes the measure of a dataset's reliability in fulfilling an organization's specific requirements. It considers factors such as accuracy, completeness, consistency, reliability, and currency.
- Data Integrity - Requires monitoring data quality across formats and over time. Data integrity in the medical device supply chain refers to the accuracy, consistency, and reliability of product data throughout its lifecycle, ensuring information remains complete and unaltered.
- Data Refresh - Act of replacing specific values or entire records of a stagnant dataset with values or records that are more current. Data refresh in the healthcare supply chain refers to the process of updating and replenishing product, usage, contract, and other data sets with the most current and accurate information.
- Data Reliability - Data reliability in the context of the healthcare supply chain refers to the accuracy, consistency, and trustworthiness of the data used to make informed decisions, track inventory, and ensure the seamless flow of medical supplies.
- Data Replication - Involves duplicating data in multiple locations to enhance availability, reliability, redundancy, and accessibility. With regards to clinical spend management analysis, data replication refers to the processes of duplicating and synchronizing healthcare expenditure and product data across multiple systems or databases to ensure data consistency and accessibility for analysis and decision making.
- Data Scalability - How well a database can handle increases or decreases in data size and complexity, while adjusting performance appropriately. Data scalability in the context of the healthcare supply chain refers to the ability of a system or platform to efficiently handle and process increasingly large volumes of healthcare expenditure data, enabling effective cost control and supply chain optimization as the organization grows or experiences higher data influx.
- Data Scrubbing - Amending or eliminating from a database data that is incomplete, inaccurate, improperly formatted, duplicated, outdated. Somewhat interchangeable with Data Cleansing. Data scrubbing in the context of clinical spend management analysis and the healthcare supply chain refers to the process of systematically cleaning and standardizing financial and procurement data to ensure accuracy and consistency, enabling more reliable cost analysis and supply chain optimization.
- Data Security - Practices that safeguard data from unauthorized access, theft, or corruption throughout entire data lifecycle. In the healthcare supply chain, refers to protection of sensitive medical and financial information through measures like encryption and access controls, ensuring confidentiality and integrity, while preventing unauthorized access or breaches.
- Data Sharing - Ability to disseminate identical sets of data resources across multiple users or applications, while maintaining data integrity. In the context of clinical spend management, refers to collaborative practice of exchanging financial and operational data among healthcare facilities, supply chains, suppliers, and other stakeholders to optimize cost efficiency and spend performance.
- Data Silo - Collection of data that isn’t readily accessible or transparent to other users or functions in a data pipeline. A data silo in the context of the healthcare supply chain refers to isolated repositories of data that aren’t easily accessible or integrated with other systems. Data silos hinder information sharing and coordination during contracting events.
- Data Stack - Suite of tools for data loading, storage, management, analysis, and business intelligence. A data stack in the healthcare supply chain refers to a structured and integrated set of technologies and tools used to collect, enrich, analyze, and visualize data to optimize spend management and efficiency of the healthcare supply chain.
- Data Standardization - Involves converting data to be analyzed to a common format for the purpose of standardization. This stage of data management assists stakeholders with making the data consistent for better analysis. If datasets aren’t standardized, they can’t be accurately compared to one another.
- Data Stewardship - In the context of the healthcare supply chain, this refers to the responsible management and governance of data throughout its lifecycle. Supply chains need data accuracy, security, and compliance for efficient and reliable operation, especially during complex medical device sourcing.
- Data Strategy - Having goals and initiatives for both the current state and the ideal future state of a data process. Establishing a framework for progressing from the current state to an optimized state. Includes consideration of organizational resources and limitations.
- Data Transfer - Exchange or sharing of information between systems or organizations. Data transfer in the medical device supply chain refers to the secure and efficient movement of electronic information, such as compliance levels, pricing trends, and usage. Transfers occur between stakeholders and systems to support optimized contract pricing and compliance.
- Data Transformation - Data transformation in clinical spend management analysis and the healthcare supply chain refers to the conversion of data from format, structure, or values of source systems into standardized, structured formats for destination systems. Data transformation focuses on improving the quality and usability of data, while data enrichment deals with expanding the breadth and depth of data. Both processes are important in preparing data for analysis.
- Data Transparency - Degree of visibility a user has into the state of data at any given point in a data pipeline. Includes expected availability and quality of that data. Data transparency in the healthcare supply chain refers to the practice of making relevant data and information easily accessible and understandable to all stakeholders, promoting visibility, accountability and informed decision making.
- Data Upload - Transmission of a data file from one system to another. In the healthcare supply chain, it refers to process of transferring electronic information or files, such as PO data, contract information, or usage records, from a local system to a centralized database or cloud platform for storage, analysis or sharing. Plays crucial role in maintaining accurate, up-to-date information for supply chain spend management.
- Data Validation - Process of verifying data accuracy and quality against predefined rules before utilizing, importing, or processing the data. In the healthcare supply chain, it refers to ensuring information entered into systems and databases is accurate, consistent, and compliant to maintain the integrity of supply chain data.
- Data Virtualization - Aggregating data from diverse systems to establish a unified, logical, virtualized data view that can be accessed by business users in real-time. Data virtualization in clinical spend analysis refers to integrating and accessing diverse data sources to facilitate real-time insights and decision-making. Sourcing analytics software can remove the manual steps and eliminate need for physical data replication.
- Data Visualization - Practice of representing data through graphics such as charts, plots and infographics for greater clarity. Visual data formats like tables, graphs and maps help supply chains build and share credible stories with clinicians for stronger PPI contract leverage.
- Data Warehouse - Central repository of information used to analyze and make informed decisions. A data warehouse in the healthcare supply chain context is a centralized repository of integrated data from various sources, including different hospital facilities and clinical product suppliers. A warehouse supports analytical and reporting processes for improving spend management, medical device procurement, and overall supply chain efficiency.
- Data Workflows - Sequence of tasks and decisions required to process a set of data. A data workflow in the healthcare supply chain is a structured sequence of data-driven tasks and processes that manage the flow of spend management information.
Data Management for Healthcare Supply Chain Has Never Been Easier
When it comes to data management for healthcare supply chains, Curvo brings serious expertise. Data enrichment and data management are at the heart of what we do for hospital systems and purchasing collectives across the United States.
Try a dashboard demo and get a feel for what data-driven sourcing can do.